SU+ Digital Repository
SU+ is an online repository for the preservation and promotion of assorted digital content at Strathmore University
Off-Campus Access to restriced resources (including the ExamsBank) now requires registration using an @strathmore.edu email address
Authentication is NOT required for On-Campus Access to content
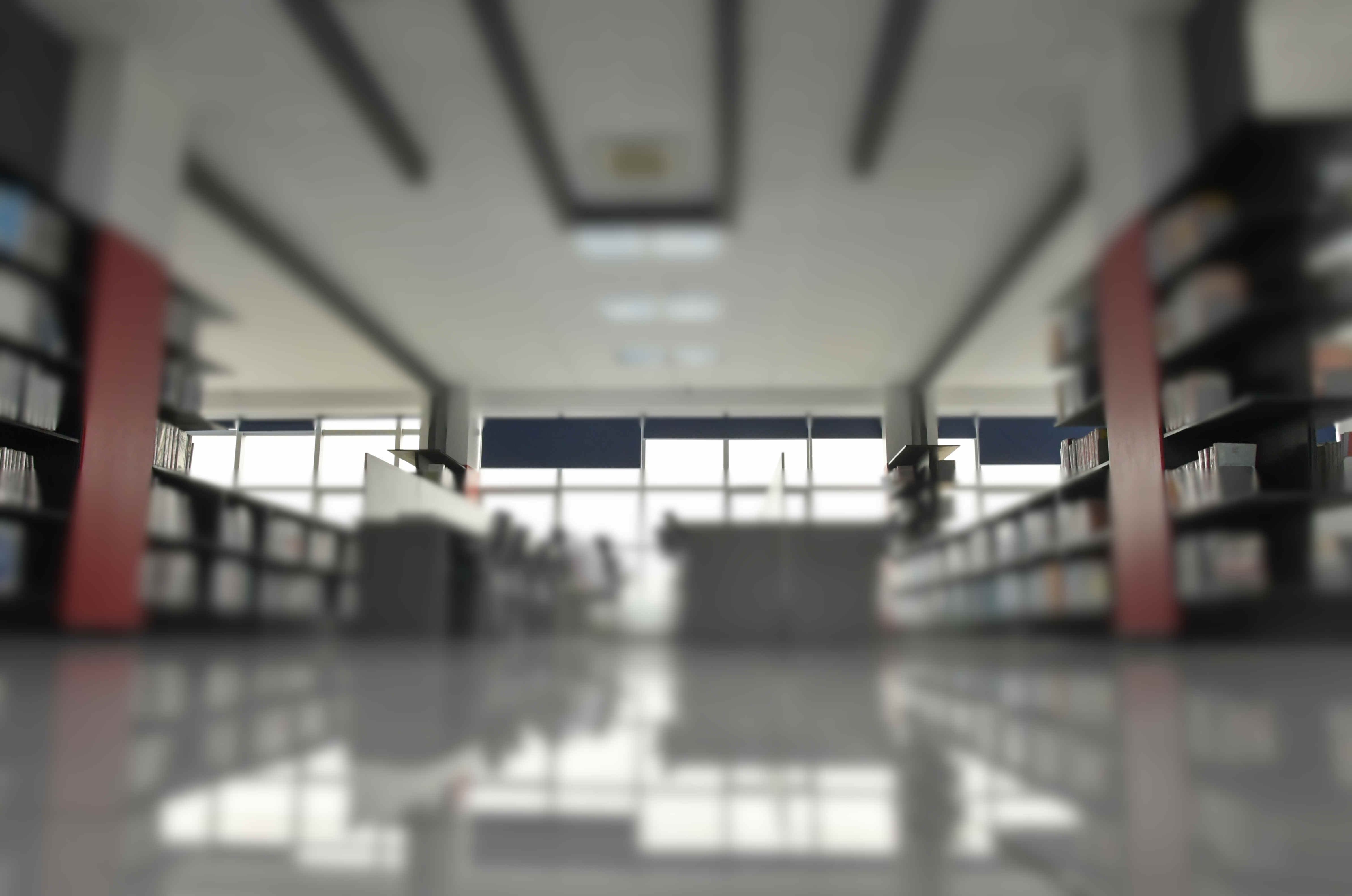
Photo by @Strathmore University
Communities in DSpace
Select a community to browse its collections.